At the same time, ATSs additionally introduce challenges similar to market fragmentation and regulatory complexities. FINRA Data supplies non-commercial use of data, particularly the ability to avoid wasting AML Risk Assessments information views and create and manage a Bond Watchlist. Upgrading to a paid membership provides you access to our extensive assortment of plug-and-play Templates designed to energy your performance—as properly as CFI’s full course catalog and accredited Certification Applications. Please bear with us as we tackle this and restore your personalized lists. Entry this and all the content on our platform by signing up for a 7-day free trial.
Whether it’s on your first residence, refinancing your current place or an funding, we have access to loans from a host of Australia’s leading lenders, one of which might be the right one for you. Finding one which’s right for you is the hard part, and that is the place we are out there in. We’ll contemplate a variety of choices to assist you get the answer you are looking for. We are particularly efficient at launching and scaling fintechs with innovative compliance strategies and delivering cost-effective managed services, assisted by proprietary regtech solutions. Get new tipps on retirement savings, investment decisions and antifraud tipps.
- It is triggered when the asset reaches a predetermined price point, allowing you to manage your money more successfully.
- The platform allows members to commerce large blocks of securities anonymously and effectively.
- The good factor about using an ATS to execute such orders is that it reduces the domino effect that enormous trades might need on the worth of an fairness.
- By incorporating clear aims, complete insurance policies, ongoing coaching, technological instruments, and regular audits, ATS operators can effectively navigate regulatory complexities and keep a robust compliance framework.
In contrast, an ATS is a decentralized venue with lighter regulatory necessities, often used for trading large blocks of securities. ATSs provide more anonymity and adaptability, which appeals to institutional buyers on the lookout for lower transaction costs and alternative trading strategies. While exchanges concentrate on transparency and market structure, ATSs supply privateness and adaptableness, serving distinct roles in monetary markets. ATS buying and selling, or Various Buying And Selling Systems, provide a different avenue for purchasing and selling securities outside traditional inventory exchanges.
Fair Access Requirements
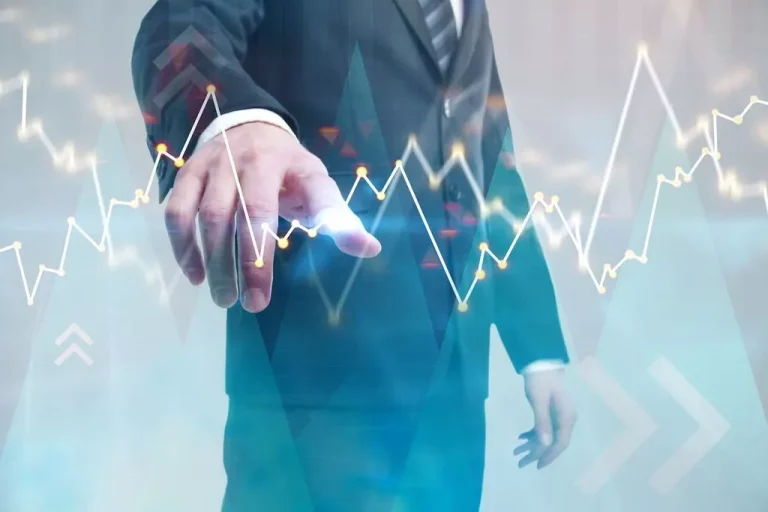
It is triggered when the asset reaches a predetermined value level, allowing you to handle your cash more successfully. In call markets, buying and selling is performed at specific instances and not repeatedly. Members place their orders, and the system matches them at predetermined occasions, usually providing better liquidity. FINRA’s Workplace of Common Counsel (OGC) staff provides broker-dealers, attorneys, registered representatives, traders, and different interested events with interpretative steering regarding FINRA’s rules.
While these platforms convey exciting alternatives for investors, understanding the regulatory landscape and exercising warning are vital to navigating this evolving landscape efficiently. Given the potential impact of ATS on the financial business, it’s essential to have correct regulation in place to safeguard market integrity and defend investors. In the United States, ATS platforms are regulated by the Securities and Change Commission (SEC) underneath the regulatory framework of Regulation ATS. The financial impacts from this Settlement might be reflected as EV customer settlement in the Company’s adjusted earnings from operations, adjusted EBITDA, adjusted net income, and adjusted fundamental earnings per share. Adjusted earnings from operations, adjusted EBITDA, adjusted web earnings, and adjusted basic earnings per share are non-IFRS monetary measures – see “Non-IFRS and Different Monetary Measures”.
#3 – Crossing Networks
In this text, we are going to dive into the definition and regulation of Different Trading Systems, shedding mild on their position and significance in today’s marketplace. Operating an Various Buying And Selling System involves adhering to strict compliance mandates to uphold truthful practices and protect buyers. These mandates are set by regulatory bodies just like the SEC underneath Regulation ATS.
Regulation
The crossing community ATS is much like the dark pool contemplating the transparency and confidentiality of the market. Nevertheless, in a crossing community https://www.xcritical.com/, the shares and securities are traded only via ATS and not by way of an exchange. Buying And Selling securities exclusively in an ATS is referred to as crossing networks. The key difference between a conventional trade and an ATS lies in their structure and regulation. Exchanges just like the NYSE or Nasdaq are centralized and closely regulated platforms promoting transparent trading and accurate price discovery.
A inventory change is a closely regulated market that brings together consumers and sellers to trade listed securities. An ATS is an electronic venue that also brings buyers and sellers together; nonetheless, it doesn’t have any regulatory obligations (though it is regulated by the SEC) and trades both listed and unlisted securities. As a end result, darkish swimming pools, along with high-frequency trading (HFT), are oft-criticized by these within the finance trade; some traders consider that these components convey an unfair benefit to certain gamers in the stock market. ATSs account for much of the liquidity found in publicly traded issues worldwide.
They provide anonymity, allowing large transactions to be executed with out revealing buying and selling intentions, which helps reduce market impact. ATSs are additionally more flexible than conventional exchanges, supporting innovative buying and selling strategies as a result of fewer regulatory constraints. They tend to be extra cost-efficient, with lower transaction fees benefiting high-volume traders. Moreover, ATSs provide entry to further liquidity pools—leading to doubtlessly higher pricing options—and can supply sooner trade execution, because of decreased operational constraints. These benefits make ATS a priceless different to traditional trading venues.
Darkish pools are designed for trading large volumes of shares with out public disclosure, whereas different ATS platforms could provide completely different benefits like decrease charges or sooner execution. ATS platforms supply greater flexibility and can be a helpful a half of a diversified trading strategy. They typically have lower fees and may execute orders more quickly than conventional exchanges. Name markets are a subset of ATS that group together orders until a specific number is reached earlier than conducting the transaction. A call market, due to this fact, determines the market-clearing value (the equilibrium worth of a traded security) primarily based on the number of securities offered and bid on by the sellers and buyers, respectively.
This permits individuals to diversify their portfolios and entry markets that is probably not obtainable on conventional exchanges. If you’re looking for alternatives to conventional stock exchanges and are considering ATS platforms, you’ll also wish to know about the most effective brokers for day buying and selling. The proper dealer could make a big distinction in your buying and selling expertise, especially when using ATS platforms. ATS platforms offer a quantity of advantages, such as decrease fees and quicker trades. Nonetheless, additionally they include their share of criticisms, primarily centered around transparency and market manipulation.